Geospatial data products in the age of AI
AI is accelerating the value and usefulness of geolocation data across several sectors - here's how.
Jul. 2, 2024
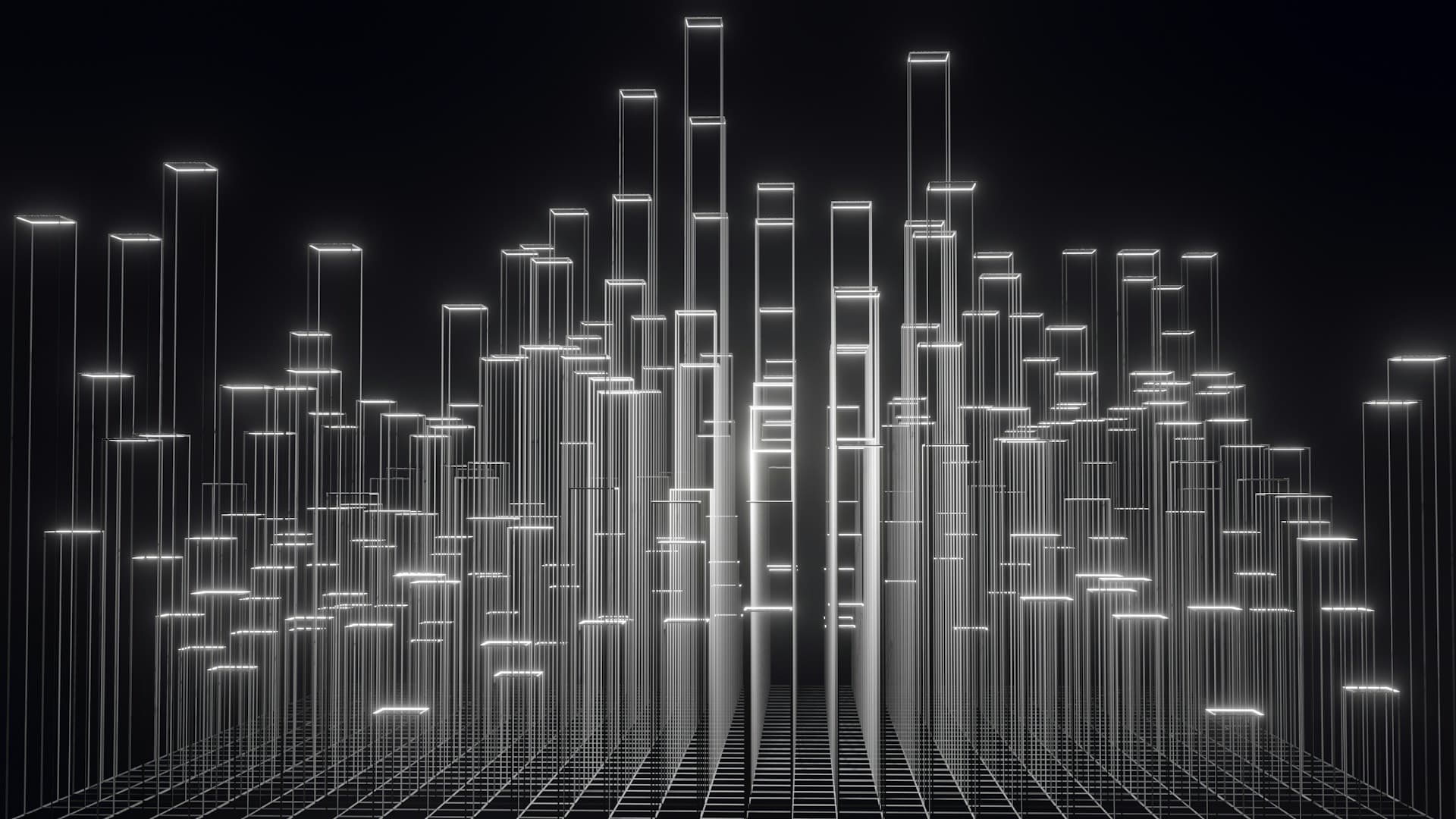
Geospatial data has historically been a challenge for businesses: expensive to collect and store, but difficult to derive value from. However, advances in AI at various stages of the data pipeline - data cleaning, image processing, and anonymisation - are giving rise to more tangible use cases for this data.
What is geospatial data?
Geospatial data is typically defined as information associated with a given location on the planet, usually a street address or a latitude-longitude pair. Geospatial data comes in many forms, each offering unique insights:
- Street Sensor Data: This includes information gathered from sensors installed on streets and public infrastructure. For example, traffic cameras and air quality monitors provide real-time data about road congestion and environmental conditions.
- Satellite Imagery: High-resolution images captured by satellites offer a bird's-eye view of the Earth's surface. These images are used for everything from tracking deforestation to monitoring urban development.
- Connected Vehicle Data: Modern vehicles equipped with GPS and IoT sensors collect vast amounts of data about travel patterns, driving behaviors, and vehicle performance. This data is crucial for optimizing traffic management and enhancing road safety.
- Smart City Sensors: Cities are increasingly deploying a network of sensors to monitor various parameters like vehicle traffic, pedestrian traffic, footfall, air quality, energy consumption, waste management, and more. These sensors provide valuable data that helps in making cities more efficient and sustainable.
The Challenge
Although this data sounds extremely useful, it's fraught with difficulties, particularly when creating revenue-generating data products. Periodic measurements associated with points in space are rarely inherently useful. The data has to be parsed, cleaned, checked for statistical anomalies or missed measurements, then analysed to be tied to a real-world metric.
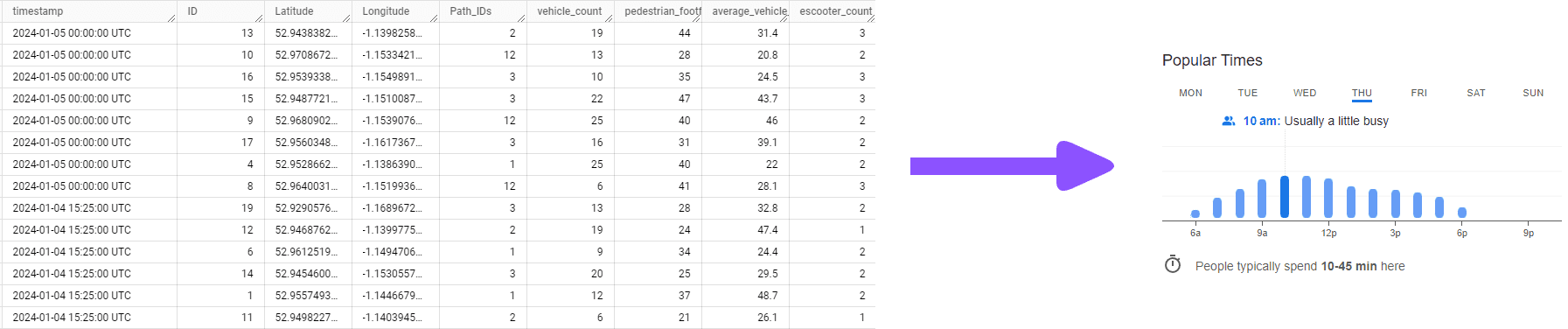
Specifically, for geospatial data:
- Data is often collected as a lat/long, and mapping it to a real-world location is non-trivial. For instance, if a we're seeing a spike of activity in a given location in the middle of a busy area in a city, how to tell which businesses in the area the visitors are actually frequenting?
- Although it doesn't look like it at first glance, geospatial data easily falls into the realm of personally identifiable information (PII). For instance, traffic sensors in less busy areas could be used to identify an individual resident's comings and goings. It's crucial to avoid these situations, especially when creating customer-facing or public data products.
- In many cases, the organisation that collects the geospatial data is not the one that processes it. An increasingly common example of this is smart cities building public mobility data portals, providing a public service to other more advanced organisations who can leverage this data to create more advanced data products. In these cases, the large volumes of raw geospatial data make the distribution of that data a key consideration and challenge.
The Opportunity
Advances in AI have enabled organisations across various sectors to tackle these challenges more efficiently, and build valuable data products faster:
AI-generated reports
Building public-facing data products is difficult, and especially so with geospatial data which is hard to communicate clearly to a broad range of users. DataPostie is working with a leading European PropTech company and a local authority to build - and in some cases monetize - public-facing AI-generated reports, turning raw geospatial data into a one-page digest that can easily be understood by a wide range of public users.
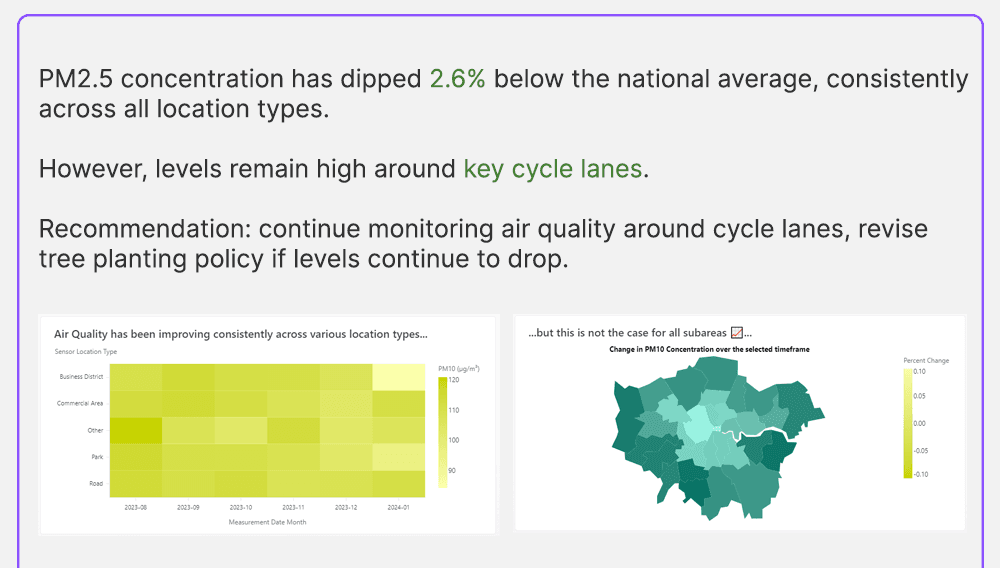
Image monetization
Many organisation also have valuable data in images, but haven't yet invested in the full AI value chain to derive insights from these images or turn them into a structured dataset. DataPostie is working with an ESG company to create a data sharing portal to share high-resolution satellite images with its partners, who then integrate them into their data value chain. As a result, this ESG company isboth generating an additional revenue stream and broadening its reach within the ESG ecosystem.
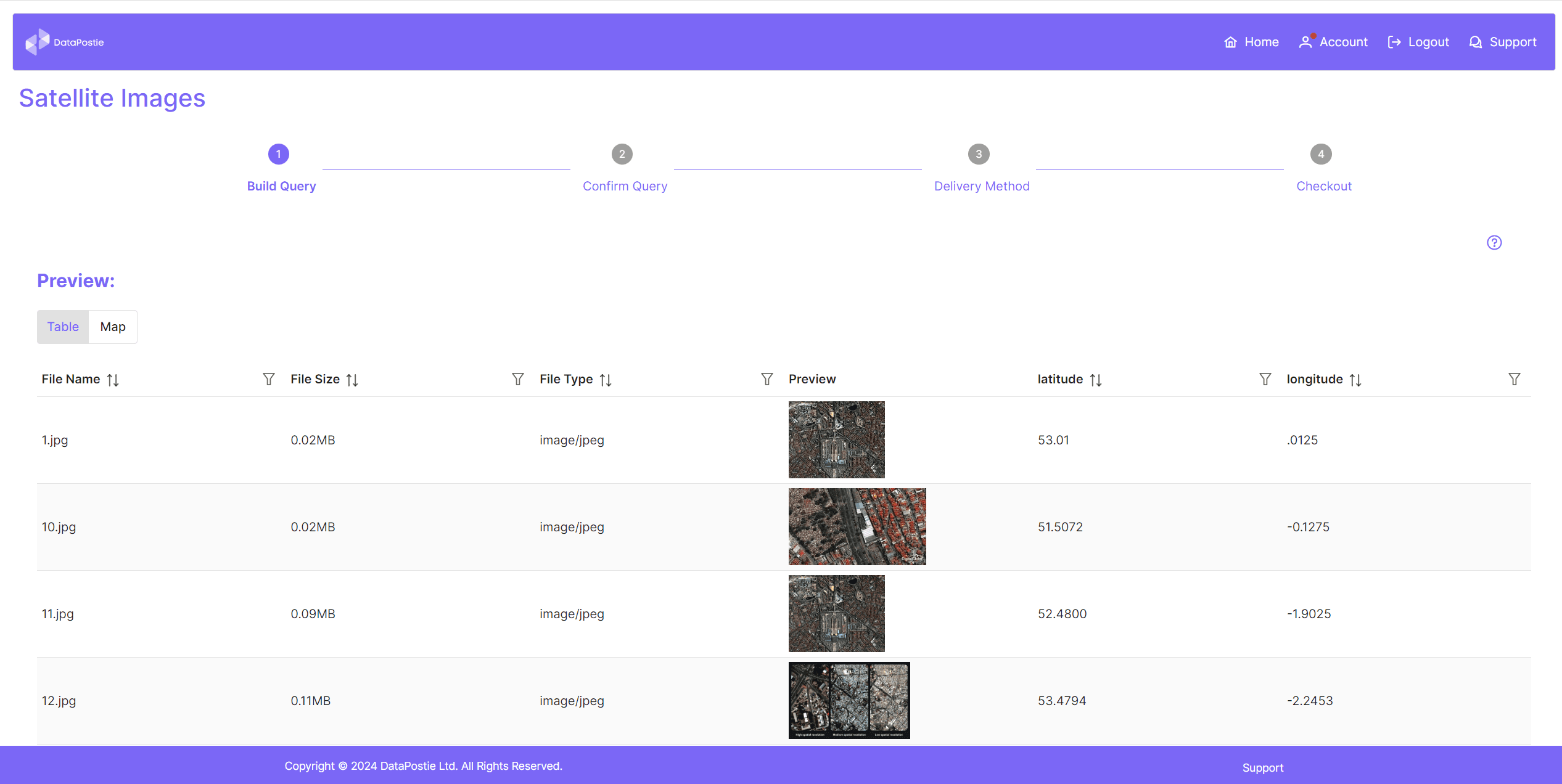
Reliable tracking
It's not just generative AI that comes into play when working with geospatial data. Companies like Spire Maritime are adding value to raw public vessel transponder data by solving an important problem for governments, insurance companies, and compliance. Vessels sometimes tamper with their coordinates, transmitting false positions through AIS data to spoof their location. Spire is using advanced algorithms to combat "vessel spoofing" and infer true vessel paths.